By mastering the art of instructing Stable Diffusion to generate images with specific body poses, we gain a deeper understanding of how to describe human posture effectively. This process, in turn, refines our ability to interpret imagery from a human perspective. While Stable Diffusion follows certain structural guidelines for prompts, it can still produce unexpected results. This discrepancy highlights the ongoing challenge of deciphering the model’s internal interpretation process.
When instructing stable diffusion to create an image, it’s important to provide clear and detailed descriptions of the desired pose. This process not only helps in generating the image but also enhances our understanding of how to communicate effectively with AI systems. It’s a learning experience that teaches us about the nuances of language and the interpretation of instructions by AI, which can sometimes yield unexpected results despite following certain guidelines. It’s a fascinating interplay between human creativity and machine learning.
Stable Diffusion
A woman bending over in the rice field planting rice seedlings etc. This is one of the most misinterpreted pose. While DALL-E 3 understands this correctly as expected, not Stable Diffusion. Is there difference in logical elaboration between the SD and DALL-E 3 ?
The differences in logical elaboration between Stable Diffusion and DALL-E 3 can be attributed to their distinct training data, design decisions, and philosophical approaches to AI tools. While both models are trained on text-image pairs and use a diffusion process to generate images, they have different strengths and styles.
Stable Diffusion tends to produce more photorealistic images but may subtly mess up details like faces. It’s also known for its ability to create detailed and complex images with a focus on realism1. On the other hand, DALL-E 3 is recognized for creating imaginative and abstract images that are less stereotypical and more aligned with the prompt1.
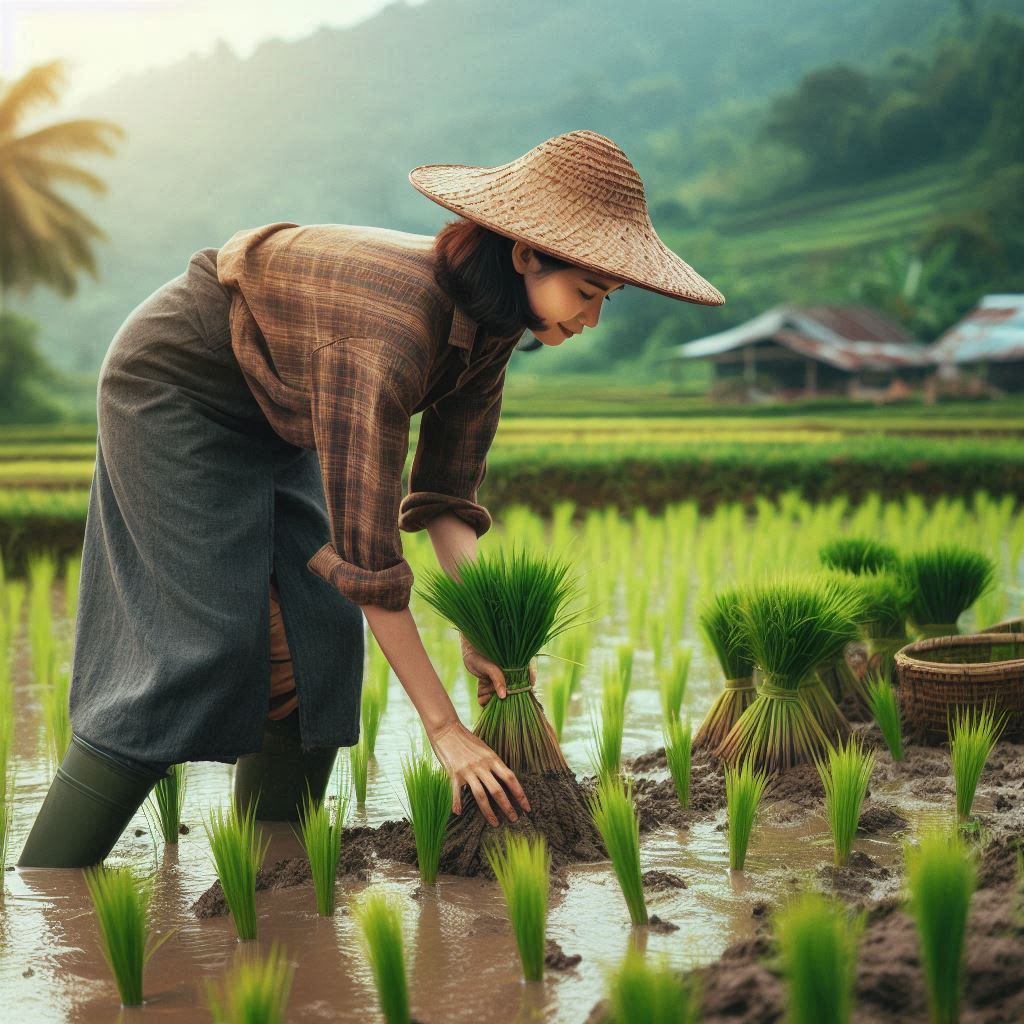
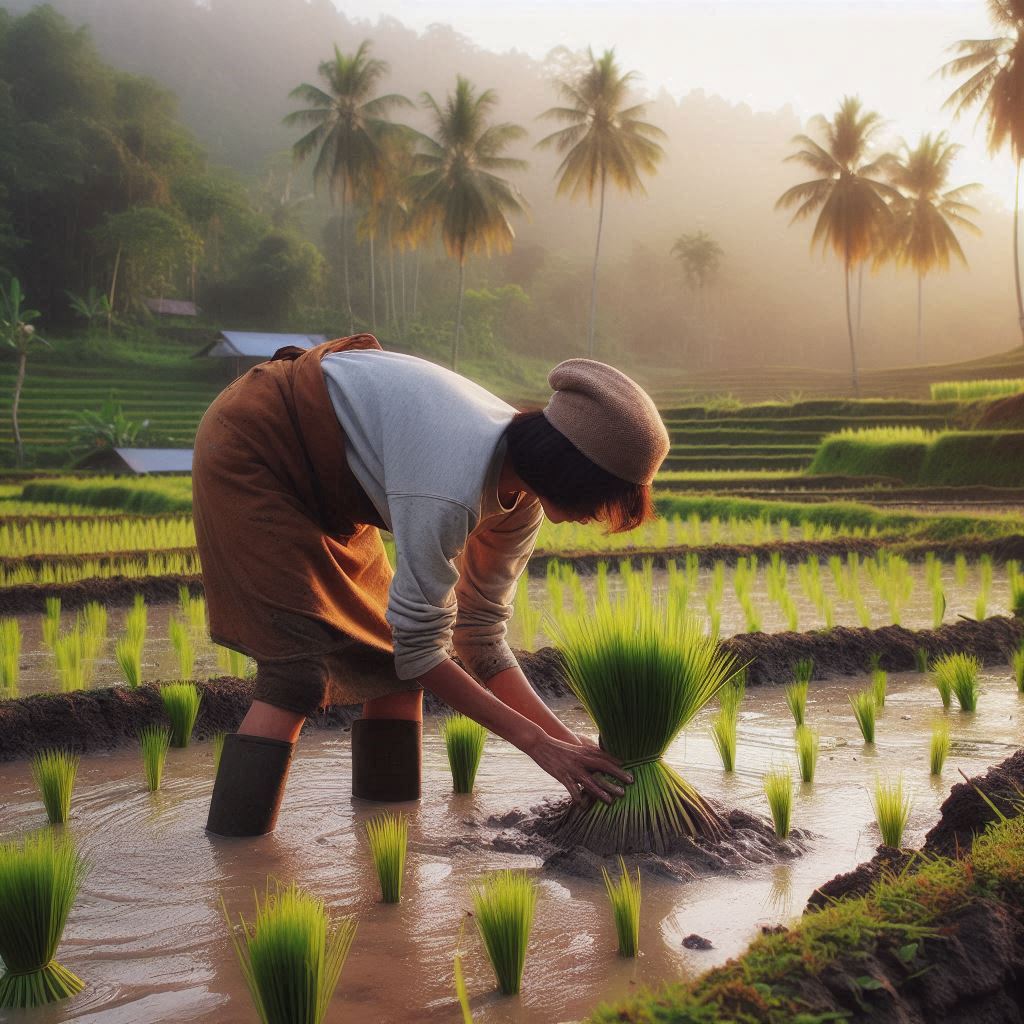
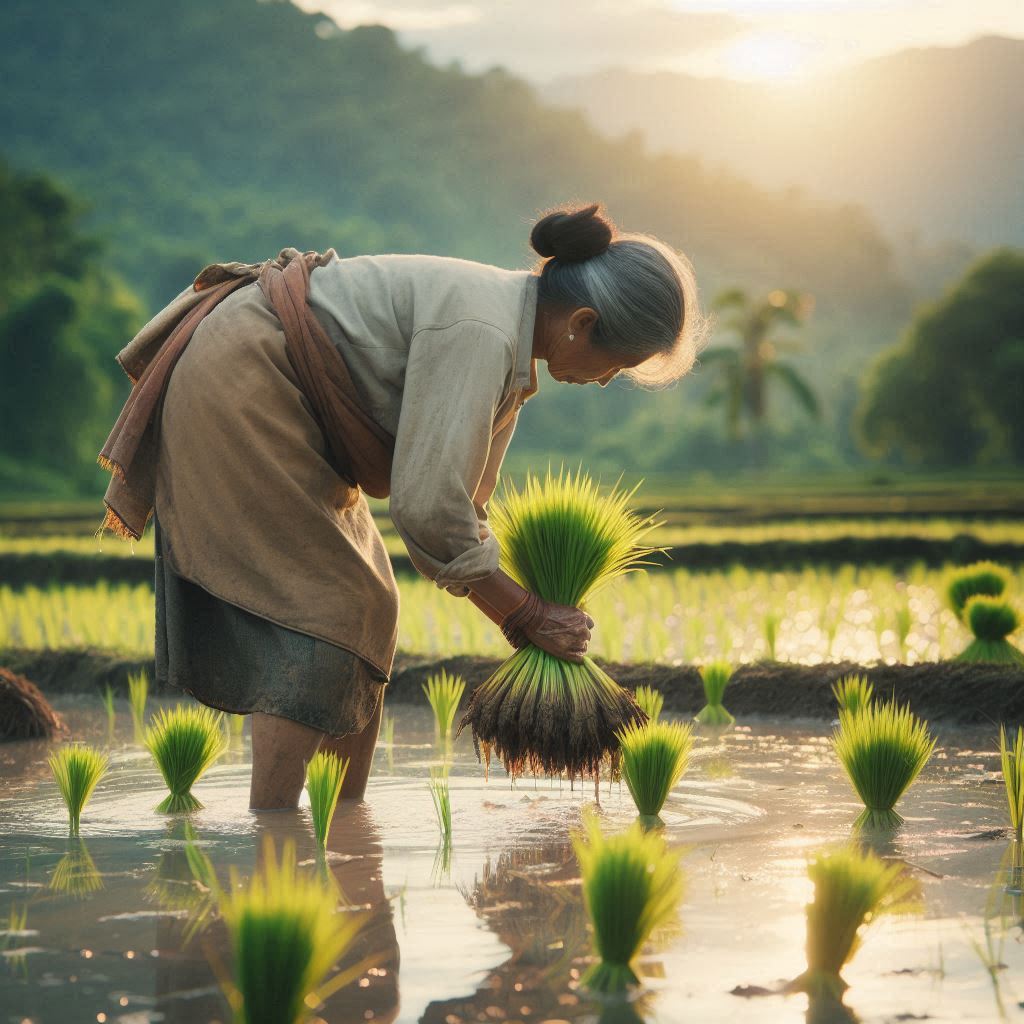
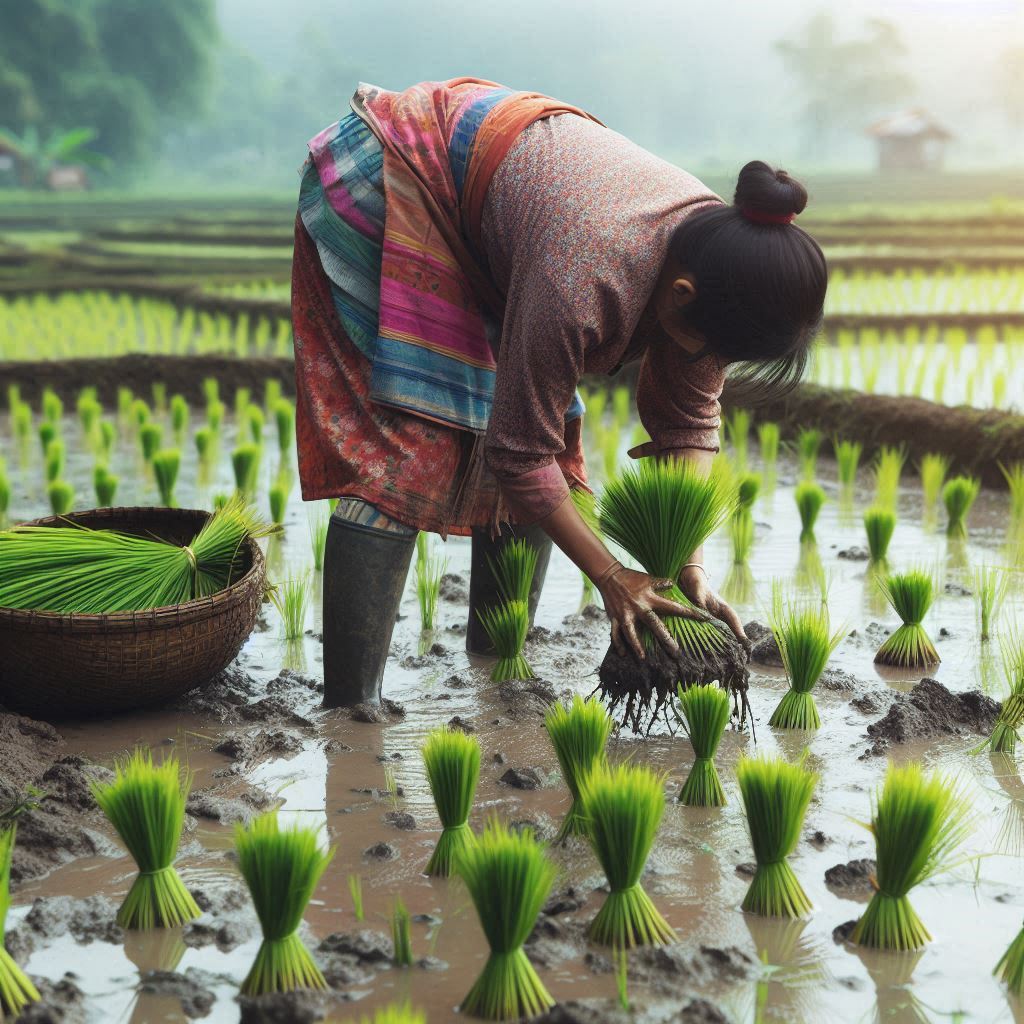
These differences mean that while both models can interpret the same prompt, they may produce different results based on their unique characteristics. It’s like comparing two artists with different styles; each has its own way of interpreting and expressing the same idea2. The choice between them would depend on the specific requirements of the task at hand, such as the need for realism versus creativity3.
What’s the meaning of diffusion in Stable Diffusion
In the context of Stable Diffusion, “diffusion” refers to a process used in deep learning models to generate images. It involves starting with random noise and gradually transforming it into a coherent image through a series of steps1. This process is inspired by the physical concept of diffusion, where particles spread out and mix evenly over time2.
Stable Diffusion uses this technique to create detailed images from text descriptions. It begins with a form of noise and then iteratively refines it into an image that matches the given prompt. The model is trained to reverse the diffusion process, effectively “denoising” the image until it resembles the desired output3. This method allows for the generation of photorealistic images and is a key component of Stable Diffusion’s ability to produce high-quality visual content1.
How different is it from DALL-E 3 ?
Stable Diffusion and DALL-E 3 are both advanced AI models for generating images from text descriptions, but they have some key differences:
- Image Style: Stable Diffusion tends to produce more photorealistic images, while DALL-E 3 often creates images that are more abstract or computer-generated1.
- Alignment: DALL-E 3 is known for being better “aligned” with the prompt, which means it may produce less stereotypical results compared to Stable Diffusion1.
- Availability: DALL-E 3 is available through specific platforms like ChatGPT and Bing Image Creator, whereas Stable Diffusion is open source and can be accessed through various apps or even installed on your own computer1.
- Philosophical Approach: The developers of Stable Diffusion and DALL-E 3 have different philosophies regarding the use of AI tools, which influences their design and implementation decisions1.
These differences mean that while both models can generate images based on the same prompt, they may do so in distinct ways, leading to different visual outcomes. The choice between them would depend on the specific needs of the task, such as whether you prefer a more realistic or abstract image style1.